Faster, more accurate diagnoses: Healthcare applications of AI research
wp:paragraph
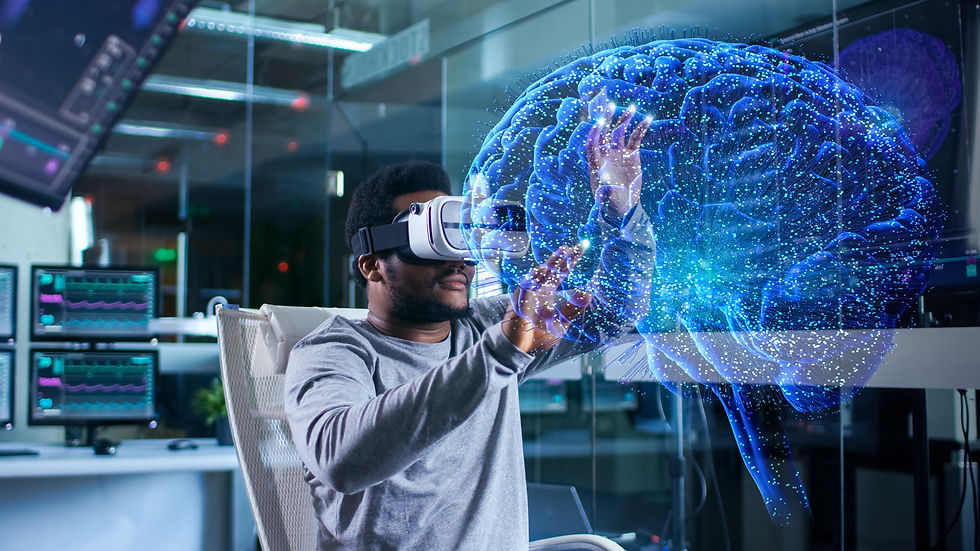
When Google DeepMind’s AlphaGo shockingly defeated legendary Go player Lee Sedol in 2016, the terms artificial intelligence (AI), machine learning and deep learning were propelled into the technological mainstream.
AI is generally defined as the capacity for a computer or machine to exhibit or simulate intelligent behaviour such as Tesla’s self-driving car and Apple’s digital assistant Siri. It is a thriving field and the focus of much research and investment. Machine learning is the ability of an AI system to extract information from raw data and learn to make predictions from new data.
Deep learning combines artificial intelligence with machine learning. It is concerned with algorithms inspired by the structure and function of the brain called artificial neural networks. Deep learning has received much attention lately both in the consumer world and throughout the medical community.
Interest in deep learning surged with the success of AlexNet, a neural network designed by Alex Krizhevsky that won the 2012 ImageNet Large Scale Visual Recognition Challenge, an annual image classification competition.
Another relatively recent advancement is the use of graphical processing units (GPUs) to power deep learning algorithms. GPUs excel at computations (multiplications and additions) needed for deep learning applications, thereby lowering application processing time.
In our lab at the University of Saskatchewan we are doing interesting deep learning research related to healthcare applications — and as a professor of electrical and computer engineering, I lead the research team. When it comes to health care, using AI or machine learning to make diagnoses is new, and there has been exciting and promising progress.
Extracting blood vessels in the eye
Detecting abnormal retinal blood vessels is useful for diagnosing diabetes and heart disease. In order to provide reliable and meaningful medical interpretations, the retinal vessel must be extracted from a retinal image for reliable and meaningful interpretations. Although manual segmentation is possible, it is a complex, time-consuming and tedious task which requires advanced professional skills.
My research team has developed a system that can segment retinal blood vessels simply by reading a raw retinal image. It is a computer-aided diagnosis system that reduces the work required by eye-care specialists and ophthalmologists, and processes images 10 times faster, while retaining high accuracy.
/wp:paragraph