When AI Becomes an Everyday Technology
- manahelthabet
- Jun 8, 2019
- 5 min read
wp:paragraph
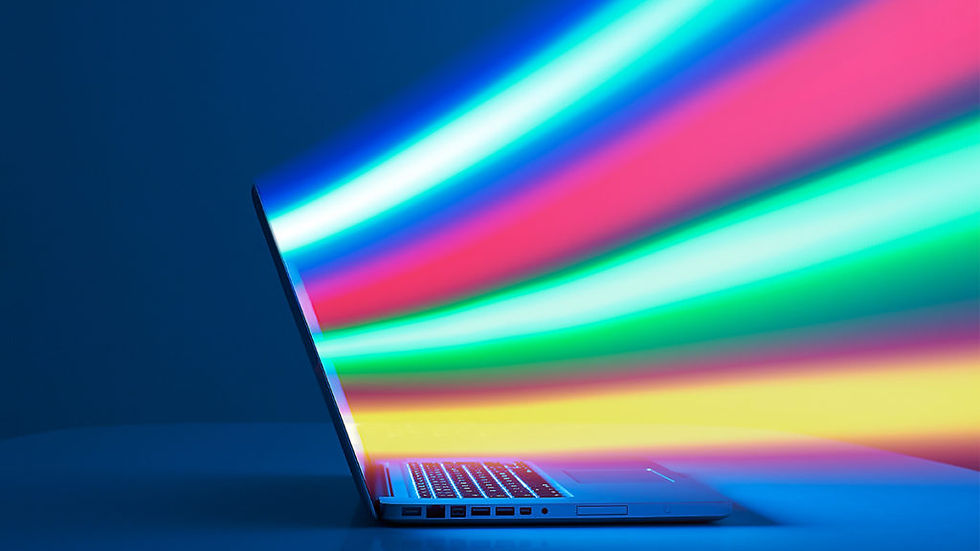
The evolution of AI has been a rich tale of exploration since its origins in the 1950’s, with the last decade providing an especially dramatic chapter of breakthrough innovations. But I believe the real story is what comes next — when the disruption stabilizes and machine learning transitions from a staple of Silicon Valley headlines to an everyday technology. It’ll be a far longer chapter — perhaps decades — in which developers all over the world use a mature set of tools to transform their industries.
In 2019, we find ourselves at the start of this new chapter. AI has undergone a remarkable refinement in recent years, as barriers to entry have fallen and a wide range of products, services, resources, and best practices have emerged. As our focus shifts — finally — from AI itself to the impact that AI can have on your business, the question is no longer how this technology works, but what it can do for you.
In other words, we’re entering the age of deployed AI. Deployed AI is about more than engineering — it’s about a shared vision. Engineering expertise will always play a role in AI. But in the age of deployed AI, our most important asset will be the vision that guides that expertise. What problems can AI solve, and what kind of data might the solution require? By what metrics will success be measured? And how can the result be integrated most effectively with the people and processes already in place in any given business? These are broad, organizational questions, and their answers won’t come from any single stakeholder. Every voice can contribute to deployed AI — technical and non-technical alike — and it’s vital that businesses establish workflows that empower everyone to play a role.
One of my favorite recent examples of this shift in possibilities comes from Carnegie Mellon University (CMU), where I formerly served as dean of the computer science department. While I was there, a student was considering her options for an upcoming artificial intelligence project, and thought of her sister, who happens to be deaf. She wanted to make it easier for her friends to learn the basics of American Sign Language, so she developed an AI-powered tool that tracked their movements and provided automatic feedback as they learned new signs. And here’s the best part: she wasn’t a computer science postdoc or even a grad student — she was a history major, taking an introductory class for fun.
It’s hard to imagine a better example of how accessible and powerful deployed AI can be — or a better indication that this technology is ready to solve problems for every business, in every industry, today.
How does deployed AI actually work? The primary characteristic is a measurable, practical impact. Simply put, a deployed AI project brings dramatic automation to a major part of your business, solving real problems for customers or employees — sometimes both — in new ways. Over the course of my career, I’ve seen countless AI projects that begin by looking for something clever to do with the data or algorithms that happen to be lying around, hoping to justify their existence in the process. In contrast, a deployed AI solution works backwards from the existing needs of the people who will use it.
So how should your own company get started identifying projects that could benefit from deployed AI? Ask yourself these questions:
How can I attract or develop the expertise needed to build the solution?
It’s vital that the members of an AI deployment team share a respect for a range of different skills. For example, imagine you’re building an AI-powered voice assistant. The project will include researchers, dialogue designers and acoustic speech modelers — among many other groups — all of whom must trust each other to solve distinct challenges intelligently. If any group feels left out, the results will range from inconsistent to downright inhumane.
How can I avoid ending up with a stranded proof-of-concept?
It’s easy to get lost in the rush of innovating, especially in a space moving as fast as AI, but it’s vital to focus on change management at the same time. This means utilizing all the traditional practices that would benefit a non-AI project: a clear north star, consistent metrics, high-quality, reliable data sets, and agility. Expect weekly reviews — at a minimum — with a continued emphasis on the end user’s experience.
Who is ultimately responsible for the decisions the AI is making?
At its core, AI is about automating judgments that have previously been the exclusive domains of humans. This is a significant challenge unto itself, of course, but it brings with it significant risk as well. Increasing effort, for instance, is required to make the decisions of AI systems more transparent and understandable in human terms. Additionally, best practices are emerging on how to use data sets and testing to ensure each sub-population of users is treated with fairness and consistency. There are also adversarial examples — deliberately misleading input intended to cause an AI system to misbehave — as well as deepfakes — realistically modified video — among many other emerging challenges. As leaders in AI, it’s our responsibility to face all of these complexities, and provide the expertise our customers and their users need to steer this technology in the right direction.
Deployed AI in action
It’s exciting to think about where deployed AI might take us as more businesses incorporate AI in their products and services. Consider some of these examples of Google Cloud AI customers that are getting creative with AI:
Global energy company AES is using drones and AutoML Vision to more safely and efficiently inspect thousands of wind turbines.
Real Estate firm Keller Williams is empowering individual realtors to work more efficiently and effectively on their own by allowing home buyers to automatically search listing photos for specific features like “granite countertops.”
The New York Times is preserving a priceless archive of millions of photos covering more than 100 years of its history. The media publication is using AI to scan and analyze images and words on thousands of archived photos.
Financial Services firm HSBC is using AI to detect fraud at the speed and scale of global commerce by screening vast amounts of customer data against publicly available data in the search for suspicious activity.
Within each of these stories, three fundamental characteristics of deployed AI can be seen in action. First, they identify a long-unsolved problem or unrealized opportunity. Next, they’re solved in a way that simply wouldn’t be possible without AI. Finally, they demonstrate that AI has a role to play in just about every industry, whether tech-focused or not.
Sooner or later, every technology transitions from an elite niche to a mainstream tool. AI is now undergoing a similar transformation. After years of hype around mysterious neural networks and the PhD researchers who design them, we’re entering an age in which just about anyone can leverage the power of intelligent algorithms to solve the problems that matter to them. Ironically, although breakthroughs get the headlines, it’s accessibility that really changes the world. That’s why, after such an eventful decade, a lack of hype around machine learning may be the most exciting development yet.
Source: https://hbr.org/2019/06/when-ai-becomes-an-everyday-technology /wp:paragraph