AI's Memory Problem
- manahelthabet
- Aug 21, 2019
- 4 min read
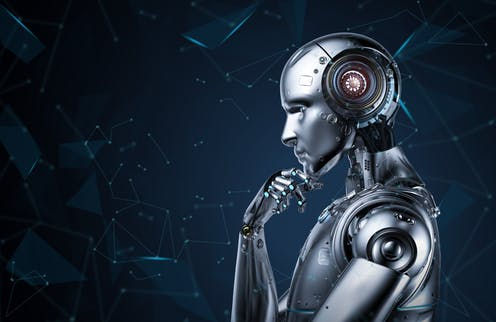
Artificial intelligence, which allows computers to learn and perform specific tasks such as image recognition and natural language processing, is loosely inspired by the human brain.
Artificial Intelligence (AI), which allows computers to learn and perform specific tasks such as image recognition and natural language processing, is loosely inspired by the human brain.
The challenge is that while the human brain has evolved over the last 3 million years, Artificial Neural Networks, the very “brain” of AI, which have only been around for a few decades and aren’t nearly as finely tuned or as sophisticated as the gray matter in our heads, are expected to perform tasks associated with human intelligence. So in our quest to create AI systems that can benefit society — in areas from image classification to voice recognition and autonomous driving — we need to find new paths to speed up the evolution of AI.
Part of this process is figuring out what types of memory work best for specific AI functions and discovering the best ways to integrate various memory solutions together. From this standpoint, AI faces two main memory limitations: density and power efficiency. AI’s need for power makes it difficult to scale AI outside of datacenters where power is readily available — particularly to the edge of the cloud where AI applications have the highest potential and value.
To enable AI at the edge, developments are being made toward domain-specific architectures that facilitate energy-efficient hardware. However, the area that will open the way for the most dramatic improvements, and where a large amount of effort should be concentrated, is the memory technology itself.
Driving AI to the Edge
Over the last half-century, a combination of public and private interest has fueled the emergence of AI and, most recently, the advent of Deep Learning (DL). DL models have — due to the exceptional perception capabilities they offer — become one of the most widespread forms of AI. A typical DL model must first be trained on massive datasets (typically on GPU servers in datacenters) to tune the network parameters, an expensive and lengthy process, before it can be deployed to make its own inferences based on input data (from sensors, cameras, etc.). DL models require such massive amounts of memory to train their many parameters that it becomes necessary to utilize off-chip memory. Hence, much of the energy cost during training is incurred because of the inefficient shuffling of gigantic data loads between off-chip DRAM and on-chip SRAM (an approach that often exceeds 50% of the total energy use). Once the model is trained, the trained network parameters must be made available to perform inference tasks in other environments.
Until recently AI applications had been confined to datacenters because of their large energy consumption and space requirements. However, over the past few years the growing demand for AI models at high scale, low latency and low cost has been pushing these applications to be run at the edge, namely in IoT and on mobile devices where power and performance are highly constrained.
This is driving a rapidly expanding hardware ecosystem that supports edge applications for inference tasks and even a nascent effort at enabling distributed training (e.g., Google’s Federated Learning models). These new architectures are primarily driven by speech recognition and image classification applications.
The growing demand combined with the increasing complexity of DL models is unsustainable in that it is causing a widening gap between what companies require in terms of energy dissipation, latency and size, and what current memory is capable of achieving. With the end of Moore’s Law and Dennard Scaling in the rear-view mirror exacerbating the issue, the semiconductor industry needs to diversify toward new memory technologies to address this paradigm shift and fulfill the demand for cheap, efficient AI hardware.
The Opportunity for New Memories
The AI landscape is a fertile ground for innovative memories with unique and improving characteristics and presents opportunities in both the datacenter and at the edge. New memory technologies can meet the demand for memory that will allow edge devices to perform DL tasks locally by both increasing the memory density and improving data access patterns, so that the need for transferring data to and from the cloud is minimized. The ability to perform perception tasks locally, with high accuracy and energy efficiency is key to the further advancement of AI.
This realization has led to significant investment in alternative memory technologies, including NAND flash, 3D XPoint (Intel’s Optane), Phase-Change Memory (PCM), Resistive Memory (ReRAM), Magneto-Resistive Memory (MRAM) and others that offer benefits such as energy efficiency, endurance and non-volatility. While facilitating AI at the edge, such memories may also allow cloud environments to perform DL model training and inference more efficiently. Additional benefits include the potential improvements in reliability and processing speed. These improvements in the memory technology will make it possible to circumvent the current hardware limitations of devices at the edge.
In particular, certain new memories offer distinct benefits due to specific inherent or unique qualities of the technology for a number of AI applications. ReRAM and PCM offer advantages for inference applications due to their superior speed (compared to Flash), density and non-volatility. MRAM offers similar advantages to ReRAM and PCM; furthermore, it exhibits ultra-high endurance such that it can compete with and complement SRAM as well as function as Flash replacement. Even at these early stages of their lifetime, these new memory technologies show enormous potential in the field of AI.
And although we are still decades away from implementing the AI we’ve been promised in science fiction, we are presently on the cusp of significant breakthroughs that will affect many aspects of our lives and provide new efficient business models. As Rockwell Anyoha writes in a Harvard special edition blog on AI, “In the first half of the 20th century, science fiction familiarized the world with the concept of artificially intelligent robots. It began with the ‘heartless’ Tin Man from the Wizard of Oz and continued with the humanoid robot that impersonated Maria in Metropolis.”
The next competitive battle is being fought in memory, and as a result, there is a tremendous amount of time, money and brain power being dedicated to figuring out how to fix AI’s memory problem. Ultimately, while these computerized brains don’t yet hold a candle to our human brains — especially pertaining to energy efficiency — it is that very uniqueness of our own minds that enables our capacity to create solutions to our many fantasies and bring artificial intelligence to life.