We Need AI That Is Explainable, Auditable, and Transparent
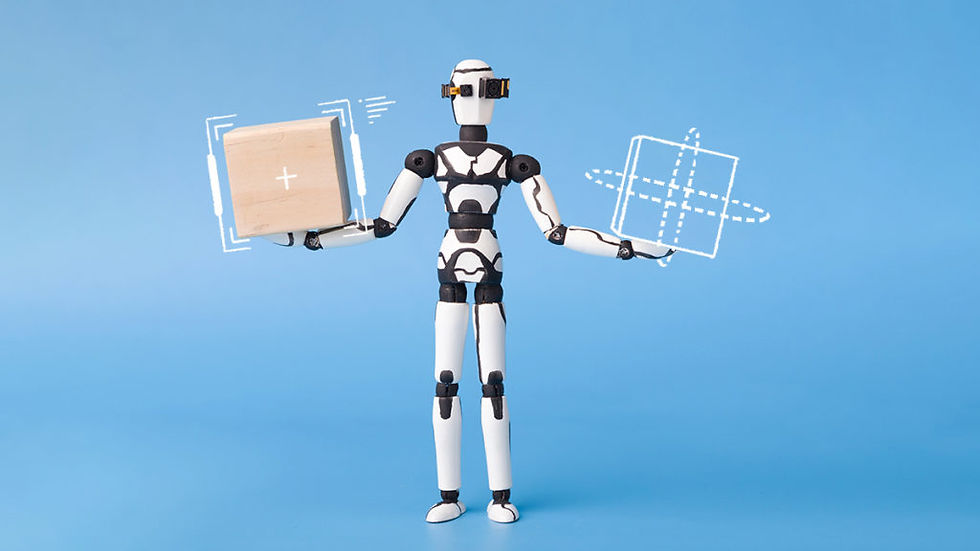
Every parent worries about the influences our children are exposed to. Who are their teachers? What movies are they watching? What video games are they playing? Are they hanging out with the right crowd? We scrutinize these influences because we know they can affect, for better or worse, the decisions our children make.
Just as we concern ourselves with who’s teaching our children, we also need to pay attention to who’s teaching our algorithms. Like humans, artificial intelligence systems learn from the environments they are exposed to and make decisions based on biases they develop. And like our children, we should expect our models to be able to explain their decisions as they develop.
As Cathy O’Neil explains in Weapons of Math Destruction, algorithms often determine what college we attend, if we get hired for a job, if we qualify for a loan to buy a house, and even who goes to prison and for how long. Unlike human decisions, these mathematical models are rarely questioned. They just show up on somebody’s computer screen and fates are determined.
In some cases, the errors of algorithms are obvious, such as when Dow Jones reported that Google was buying Apple for $9 billion and the bots fell for it or when Microsoft’s Tay chatbot went berserk on Twitter — but often they are not. What’s far more insidious and pervasive are the more subtle glitches that go unnoticed, but have very real effects on people’s lives.
Once you get on the wrong side of an algorithm, your life immediately becomes more difficult. Unable to get into a good school or to get a job, you earn less money and live in a worse neighborhood. Those facts get fed into new algorithms and your situation degrades even further. Each step of your descent is documented, measured, and evaluated.
Consider the case of Sarah Wysocki, a fifth grade teacher who — despite being lauded by parents, students, and administrators alike — was fired from the D.C. school district because an algorithm judged her performance to be sub-par. Why? It’s not exactly clear, because the system was too complex to be understood by those who fired her.
Make no mistake, as we increasingly outsource decisions to algorithms, the problem has the potential to become even more Kafkaesque. It is imperative that we begin to take the problem of AI bias seriously and take steps to mitigate its effects by making our systems more transparent, explainable, and auditable.
Sources of Bias
Bias in AI systems has two major sources: the data sets on which models are trained, and the design of the models themselves. Biases in the data sets on which algorithms are trained can be subtle, for example, such as when smartphone apps are used to monitor potholes and alert authorities to contact maintenance crews. That may be efficient, but it’s bound to undercount poorer areas where fewer people have smartphones.
In other cases, data that is not collected can affect results. Analysts suspect that’s what happened when Google Flu Trends predicted almost double as many cases in 2013 as there actually were. What appears to have happened is that increased media coverage led to more searches by people who weren’t sick.
Yet another source of data bias happens when human biases carry over into AI systems. For example, biases in the judicial system affect who gets charged and sentenced for crimes. If that data is then used to predict who is likely to commit crimes, then those biases will carry over. In other cases, humans are used to tag data and may direct input bias into the system.
This type of bias is pervasive and difficult to eliminate. In fact, Amazon was forced to scrap an AI-powered recruiting tool because they could not remove gender bias from the results. They were unfairly favoring men because the training data they used taught the system that most of the previously-hired employees of the firm that were viewed as successful were male. Even when they eliminated any specific mention of gender, certain words which appeared more often in male resumes than female resumes were identified by the system as proxies for gender.
A second major source of bias results from how decision-making models are designed. For example, if a teacher’s ability is evaluated based on test scores, then other aspects of performance, such as taking on children with learning differences or emotional problems, would fail to register, or even unfairly penalize them. In other cases, models are constructed according to what data is easiest to acquire or the model is overfit to a specific set of cases and is then applied too broadly.
Overcoming Bias
With so many diverse sources of bias, we do not think it is realistic to believe we can eliminate it entirely, or even substantially. However, what we can do is make our AI systems more explainable, auditable, and transparent. We suggest three practical steps leaders can take to mitigate the effects of bias.
First, AI systems must be subjected to vigorous human review. For example, one study cited by a White House report during the Obama administration found that while machines had a 7.5% error rate in reading radiology images, and humans had a 3.5% error rate, when humans combined their work with machines the error rate dropped to 0.5%.
Second, much like banks are required by law to “know their customer,” engineers that build systems need to know their algorithms. For example, Eric Haller, head of Datalabs at Experian told us that unlike decades ago, when the models they used were fairly simple, in the AI era, his data scientists need to be much more careful. “In the past, we just needed to keep accurate records so that, if a mistake was made, we could go back, find the problem and fix it,” he told us. “Now, when so many of our models are powered by artificial intelligence, it’s not so easy. We can’t just download open-source code and run it. We need to understand, on a very deep level, every line of code that goes into our algorithms and be able to explain it to external stakeholders.”
Third, AI systems, and the data sources used to train them, need to be transparent and available for audit. Legislative frameworks like GDPR in Europe have made some promising first steps, but clearly more work needs to be done. We wouldn’t find it acceptable for humans to be making decisions without any oversight, so there’s no reason why we should accept it when machines make decisions.
Perhaps most of all, we need to shift from a culture of automation to augmentation. Artificial intelligence works best not as some sort of magic box you use to replace humans and cut costs, but as a force multiplier that you use to create new value. By making AI more explainable, auditable and transparent, we can not only make our systems more fair, we can make them vastly more effective and more useful.